Machine learning tool slowed the spread of COVID in Greece
Greece’s algorithm-informed testing system for international travelers helped the country keep its borders open while protecting public health despite scarce testing resources
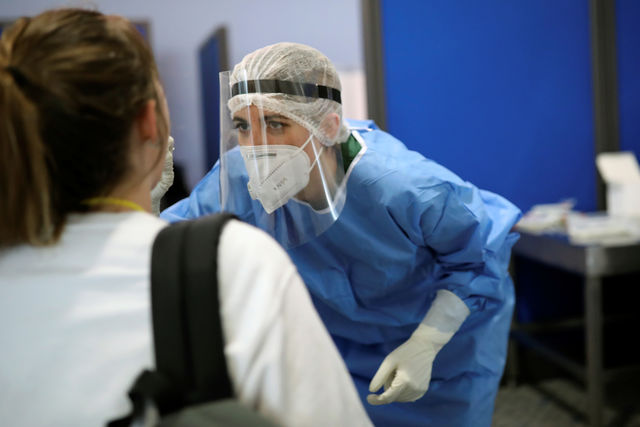
In the summer of 2020, as countries around the world sealed their borders to stem the spread of COVID-19, Greece took a different path. Eager to both protect the health of its citizens and maintain the flow of tourists into the country, Greece launched what is believed to have been the first effort to harness machine learning to reduce the spread of an infectious disease.
The effort, a collaboration between machine learning experts and epidemiologists, provided the Greek government with real-time estimates of COVID-19 prevalence among travelers entering the country, helping inform testing protocols and direct scarce testing resources towards those most likely to be infected. This allowed authorities to identify about 40 percent of infected but asymptomatic travelers, roughly double the number that would have been identified through random testing. The initiative also helped the country manage its scarce testing resources while at the same time minimize the entry of asymptomatic cases, helping reduce the spread of COVID and save lives.
“While machine learning has great potential for health care applications, public health leaders have been slow to explore potential applications,” said Hamsa Bastani, Assistant Professor of Operations, Information, and Decisions at the Wharton School at the University of Pennsylvania, and one of the machine learning experts who constructed the model and designed and coded the algorithm. “That is because the stakes are high when you are talking about health and machine learning [which] is sometimes seen as a black box and not transparent. There is also, rightly, a high value placed on human observation in health care. We know that physicians can observe things and make important inferences that machines cannot. But COVID-19 helped reduce the barriers of entry and convinced health officials that machine learning was a tool worth trying.”
The algorithm, dubbed “Eva” by researchers, adapted and adjusted its recommendations on which travelers should be tested, as COVID rates shifted in travelers’ countries of origin. It also took into account the delay between infection and symptoms and the typical COVID infection cycle that sees exponential increases in cases over several weeks, followed by a steep decline and a plateau, and then an increase back to exponential levels.
The effort relied on collecting key demographic information from travelers 24 hours before they traveled, including their country, region, age, and gender. This data was then integrated with testing results from previous travelers with similar demographics, and data on current infection rates in countries of origin to determine if travelers should be tested upon arrival and self-isolate while their test results were being processed. Travelers who tested positive were quarantined and contact tracing initiated.
“Our team included attorneys and privacy experts who helped us ensure we only collected the minimum information from travelers,” said Bastani. “It was surprising to us all how little information was needed to get powerful results. For example, we didn’t have to ask for a traveler’s occupation or travel itinerary.”
By comparing Eva’s performance against modeled counterfactual scenarios, researchers estimate that Eva identified 1.85 times as many infected but asymptomatic travelers as random surveillance testing, and two to four times as many during peak travel. The algorithm also identified 1.25-1.45 times as many infected travelers as testing protocols that utilize only epidemiological metrics.
The researchers concluded in an article recently published in Nature “…our work represents a successful example of the potential of reinforcement learning and real-time data for safeguarding public health.”
The researchers believe that Eva was the first large-scale, data-driven system designed and deployed during the COVID-19 crisis, and they attribute its success to a few key features:
· Eva’s algorithm was designed to both minimize the amount of personal data collected and protect privacy
· Eva prioritized interpretability and transparent reasoning to ensure all partners trusted the system’s recommendations
· Eva was designed for flexibility to ensure the system could be updated as needs and circumstances shifted
Eva was operational from August through November 2020, at a time when other European countries had launched a dizzying array of strategies to reduce the importation of COVID-19 cases. Some countries allowed only travelers from designated “whitelisted” countries. Others required travelers from designated “grey list” countries to provide proof of a negative RT-PCR test before arrival. Others required travelers from “red list” countries to quarantine upon arrival. And others forbade any non-essential travel from “blacklisted” countries. Most countries utilized a combination of these strategies.
The result was not only confusion, it was also often illogical and ineffective. For example, as of July 1st, 2020, Spain allowed unrestricted travel from a small group of countries while many of those same countries were required to quarantine upon arrival in fellow European Union member state Croatia. Few of these strategies took into account that a country’s COVID cases typically spike nine days before the increased infection rates are reflected in public data – meaning they were typically responding to pandemic surges more than a week late.
Eva’s targeting was most effective at a time like the summer of 2020, when testing resources were scarce, and in a country like Greece, that could maintain a central database for immigration and testing results. Countries with the resources to test all incoming travelers (e.g., with rapid testing kits) or who do not maintain centralized databases of testing results would experience little benefit from Eva.
Algorithms are already commonly used by online advertisers and social media companies. The researchers involved in this initiative hope the success of Eva will pave the way for machine learning to be harnessed for other public health concerns including clinical trials, where it holds the potential to accelerate research.