AI powering a revolution in identifying the poorest and most vulnerable for help
Advances in the technology are helping governments use satellite images, phone records, and other new data sources to better target health, poverty, and humanitarian interventions
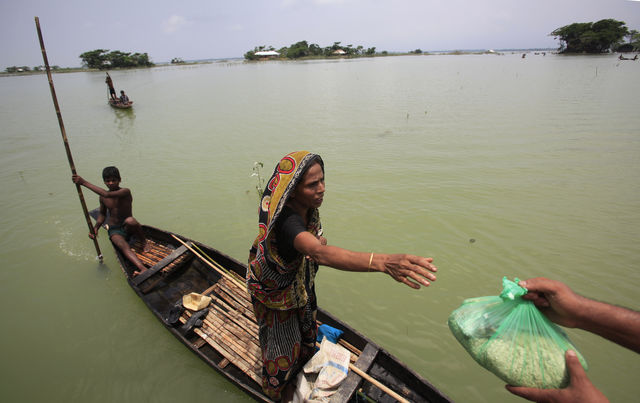
During the pandemic, leaders around the world harnessed recent innovations in artificial intelligence (AI) to search satellite images and phone records, and quickly and effectively identify the poorest families in need of emergency social protections – sparking a revolution in targeting aid at scale.
“For more than a decade there has been a long line of academic work using phone data and satellite imagery to infer poverty levels,” said Emily Aiken at the University of California Berkeley’s Center for Effective Global Action. “The pandemic forced a revolution in this space. Government leaders wanted to roll out social protection programs but didn’t have data to locate those in need. They harnessed the academic work and the field leapt forward.”
AI-supported identification of the poorest of the poor at scale is one of a range of innovations, including mRNA vaccines and telehealth, propelled forward by the pandemic. Many of these innovations promise even greater impact post-pandemic.
Previously, governments interested in targeting programs relied on labor-intensive door-to-door surveys. Dr. Mushtaque Chowdhury, former vice chair of BRAC, recalled his organization’s early efforts to provide destitute families with relief during massive flooding shortly after Bangladesh gained independence 50 years ago. “We sent people door-to-door and asked people, where can we find the family in greatest need,” he said. “People were very honest. They would tell us, ‘We don’t have much, but there is a family over that hill who is even worse off.’ And that is how we stretched our funds to have the greatest impact.”
During the pandemic, social distancing requirements and lockdowns made such interactions dangerous and the urgent need for aid ruled out time-consuming national surveys. Many existing government social registries were not up-to-date and, according to the World Bank, roughly half of low-income countries have not conducted a census in the past 10 years, and 18 percent have not done so in the past 20 years.
To find the families most in need, leaders in Togo, the Democratic Republic of Congo, Colombia, Malawi, and Liberia launched ambitious high-tech projects that harnessed phone and satellite data, and new machine learning and geospatial analysis innovations to interpret data.
Leaders in Togo worked with Aiken and her colleagues at the UC Berkeley’s Center for Effective Global Action to develop machine learning algorithms capable of scouring satellite images and cellphone data for telltale signs of extreme poverty such as thatched roofs, unpaved roads, and mobile phone calling patterns and credit purchases made in smaller increments. They calibrated their analysis using a 2018 government household survey that had reached only part of the country and a phone survey of a poor rural district.
Within two weeks of launch, 30,000 of Togo’s poorest people, many of them in remote areas, had received emergency cash payments. Eventually the satellite imagery and phone data helped aid reach 138,000 poor rural people. The program encouraged women’s enrollment by providing men who enrolled the equivalent of US$18.12, whereas women enrolling received the equivalent of US$21.14.
The Democratic Republic of Congo’s leaders, working with the DRC Social Fund and the Solidarité par Transferts Economiques contre la Pauvreté à Kinshasa program used AI analysis of satellite imagery to identify flood-prone areas. Everyone living in those areas, which are almost universally the poorest of the poor, was deemed eligible for the country’s COVID-relief aid. The government then obtained mobile phone numbers of everyone living in those areas and set up a selfregistration system for beneficiaries.
Likewise, leaders in Liberia used satellite data to identify communities with overlapping vulnerabilities – high population density, flood prone, and poor water and sanitation access. Everyone living in those areas was eligible for COVID relief.
Leaders in Colombia used phone data, combined with credit scores and an existing social registry, to identify families for COVID-related cash transfers. Leaders in Malawi used similar sources of data as the Togo effort to identify the poorest neighborhoods for COVID-relief cash transfer programs.
Already, these tools are being used beyond the pandemic. Atlas AI, a public benefit corporation funded in part by the Rockefeller Foundation, is supporting the development of a data science sector for social impact, such as using satellite imagery and other data to develop high-resolution datasets on wealth, consumption, and agricultural yields for policymakers.
“We can go kilometer by kilometer across countries to identify vulnerabilities,” said Vivek Sakhrani, head of applied data science at Atlas AI. “We can estimate how many girls ages 14-20 live in a certain area for education programs or how many women of childbearing age may need maternal health support. We can identify what people are growing in a given area and what their harvest are like. We have data on daily spending that allows us not just to know that people in a certain area live under the global poverty line of US$2 per person a day, but how much they actually spend per day.”
He added, “Digital impressions of human activity, with the right framework, and partnerships can help us understand far more about the challenges communities face.”
Atlas AI, meanwhile, also partnered with a local organization in Ghana to help identify families who would benefit most from subsidies for water access. Atlas AI is currently providing GiveDirectly with data on the poorest areas across 50 countries. The data doesn’t identify individuals or names, but does identify the poorest regions, villages, and neighborhoods to help target programming. Atlas AI is also working with health and infrastructure experts to measure the travel time required to reach health facilities in Kenya and other parts of Africa to help prioritize vaccine deliveries and nutritional supplement programs.
“The potential for impact is huge,” said Sakhrani. “And what has changed the game is that I can run these analyses on my laptop in a way that I could not three to four years ago… This unlocks benefits for the global community because we can move to respond to disasters and emergencies so much faster.”
Malawi’s leaders, partnering with Digital Impact Alliance for Health, have also leveraged cell data to determine the optimal placement of new health facilities.
Leading machine learning experts in Africa have founded the Deep Learning Indaba, an organization working to strengthen and promote the development of machine learning and artificial intelligence expertise for social impact across the continent.
And Facebook Disaster Maps is helping governments and aid agencies utilize information about the social network platform usage in areas impacted by natural disasters, to identify who has been displaced and where they have moved.
The new AI-assisted targeting approach, though faster and cheaper than national surveys, should not replace them, cautioned Aiken. “If you can go into the field to get ground truth, you should do that because it is more accurate. Governments should return to in-field data collection and use satellite and phone data to complement the field data during emergencies or disasters.”
An analysis of the Togo initiative found the machine-learning approach increases exclusion errors by nine to 35 percent compared to a hypothetical door-to-door social protection registry (which does not yet exist in Togo). The Center for Global Development has produced a paper with key takeaways from high-tech approaches to COVID-relief efforts.
Exemplars in Global Health research has also demonstrated the importance of precisely targeting cash transfer programs, such as Peru’s conditional cash transfer program Juntos, and the Kyrgyz Republic’s Universal Monthly Benefit program, both of which supported the poorest families to reduce childhood stunting.
How can we help you?
Exemplars in Global Health believes that the quickest path to improving health outcomes to identify positive outliers in health and help leaders implement lessons in their own countries. With our network of in-country and cross-country partners, we research countries that have made extraordinary progress in important health outcomes and share actionable lessons with public health decisionmakers. Our research can support you to learn about a new issue, design a new policy, or implement a new program by providing context-specific recommendations rooted in Exemplar findings. Our decision-support offerings include courses, workshops, peer-to-peer collaboration support, tailored analyses, and sub-national research. If you'd like to find out more about how we could help you, please click here. Please also consider registering for our platform and signing up for our monthly newsletter so you never miss new insights from Exemplar countries. You can also follow us on Twitter and LinkedIn. |