After identifying Exemplar countries in stunting reduction with our Technical Advisory Group (TAG), we took a holistic approach to analyze the stunting reduction story in each country, adapting our methods based on the data available. Our learnings were synthesized from four methods of inquiry:
- Literature review
- Quantitative analysis
- Qualitative analysis
- Policy / program review & financing analysis
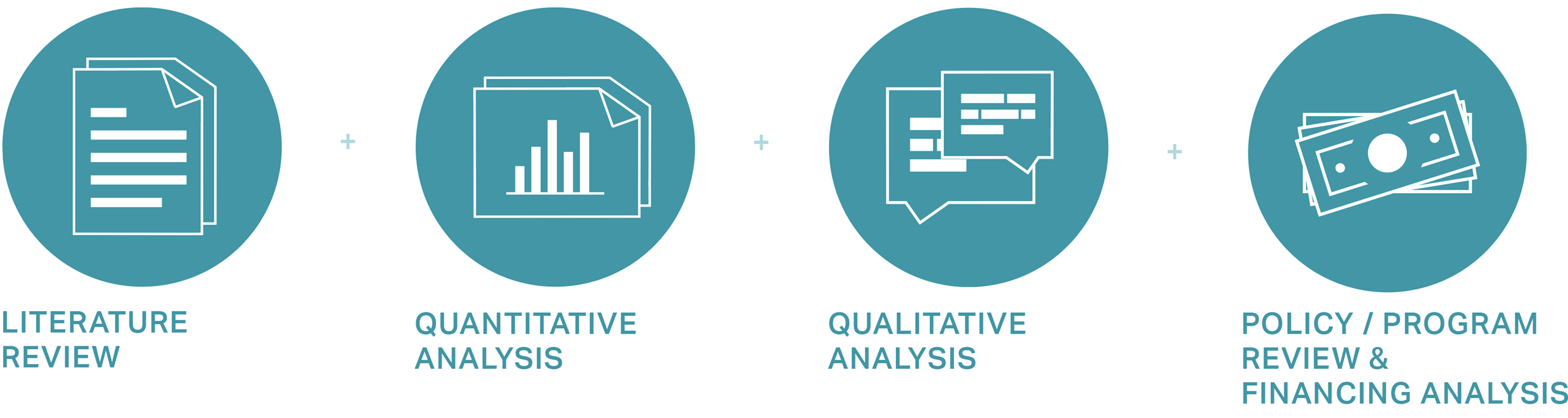
Literature review
We initiated our research process with a systematic literature review of contextual factors, national and subnational interventions, policies, strategies, programs, and other initiatives that may have contributed to reductions in child stunting over time. Broad searches were followed by de-duplication and predefined exclusions.
The graphic below summarizes this process for Peru, one of our Exemplar countries.
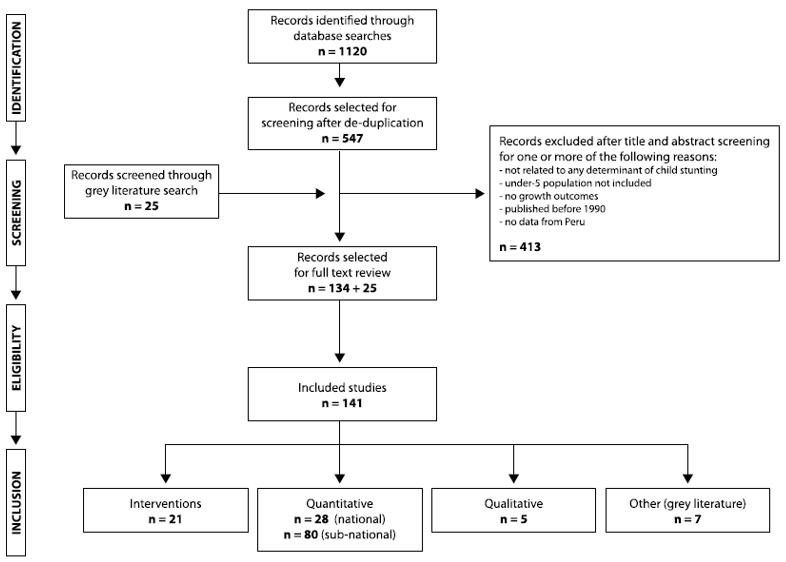
Quantitative analysis
Our quantitative methods spanned two primary categories of analysis:
- Descriptive analyses to provide contextual understanding of the stunting decline across geographic, socioeconomic, gender, and age segments.
- Linear mixed effect regressions (or, based on data availability, linear multivariable regression-based difference-in-difference analysis) and regression-based decomposition analyses to understand the major predictors of the stunting decline, as well as their relative importance to each country’s progress. We utilized multiple data sources in each country ranging from Demographic and Health Surveys (DHS), other household surveys (MICS), census data, and data from specific programs, at the household, ecological (subnational-level), and national levels.
We organized available variables for analysis into a conceptual framework adapted from the UNICEF / Lancet nutrition series undernutrition conceptual framework, which groups determinants of stunting into their causal hierarchical levels.
UNICEF / Lancet nutrition series undernutrition conceptual framework
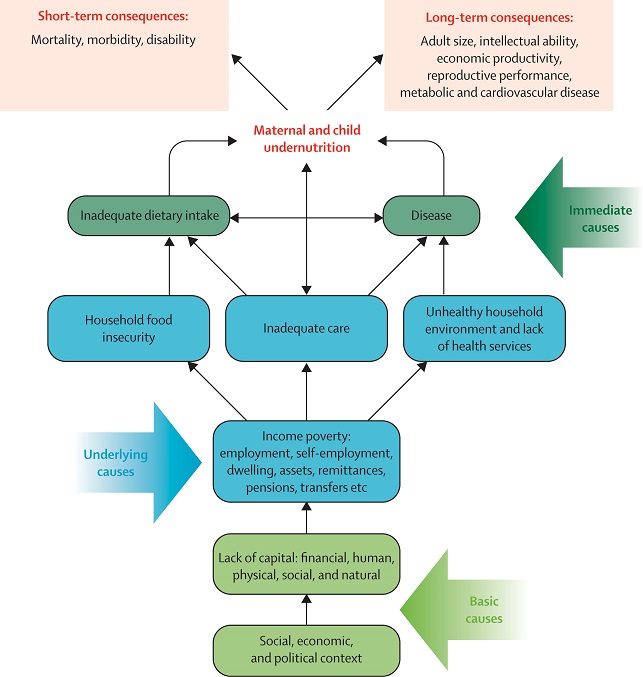
Descriptive analysis
To explore geospatial within-region stunting variation, we utilized five-by-five kilometer area stunting estimates modeled by the Institute of Health Metrics and Evaluation (IHME). IHME uses available DHS and MICS datasets incorporated into Bayesian spatial models to generate posterior predicted prevalence of stunting. The model draws strength from covariables, years, and locations where data is available.
To examine inequalities across population subgroups, we looked at stunting, as well as coverage of relevant interventions, across important subnational dimensions: wealth quintile, level of maternal education, area of residence (urban or rural), child gender, and wealth quintile by area of residence.
Finally, to examine the growth faltering process from birth to five years of age, we estimated child growth curves also known as Victora curves. Smoothed polynomial curves were used to plot predicted child height-for-age Z-scores against child age to assess growth trajectories and gain an understanding of how stunting risk changes with age.
Linear regression models
Where there was sufficient subnational ecological data over our study period, we conducted a linear mixed effects regression. The linear mixed effects regression is a time-series ecological analysis using region-year data (i.e., each data point is a region-level estimate for one given year) and two-year lagged stunting prevalence as the outcome.
We used a hierarchical modeling approach in line with our adapted conceptual framework to account for the mediation of distal factors by proximal causes. The model accounted for the fixed effects of predictors and time; the random effects account for variability within and between states, as well as over time. Finally, we included a two-year time lag between predictive variables and outcome variable (stunting prevalence), given the time necessary for interventions and other factors to make an impact. We modeled at the region-level to counteract individual characteristics (especially poverty) which are not well measured in surveys and can result in confounding (i.e., any determinant associated with poverty is identified as a predictor of stunting). However, this methodology makes inferences susceptible to ecological fallacy (i.e., correlations between variables at the group level do not always translate into correlations at the individual level).
Where there was not sufficient subnational ecological data over our study period, we instead undertook a linear multivariable regression based on a difference-in-difference analysis framework. This approach is used to evaluate if a change in a proposed predictor of HAZ leads to a change in HAZ over the studied time period.
To examine the association between HAZ and various indicators, we conducted a series of step-wise linear regression models. A hierarchical modelling approach using distal, intermediate and proximal level variables was executed as suggested by Victora 1997 to generate the final multivariable models. Variables within each level were selected from our general conceptual framework.
Step 1 was a series of bivariate regressions to determine crude associations between indicators in our conceptual framework and HAZ outcome. Step 2 was to use all candidate variables for multivariable model building (i.e., with p-value ≤0.20) irrespective of their direction to move forward for multivariable modeling. Bivariate correlations estimate the absolute crude associations between the covariable and the outcome, and they highlight the total (unadjusted) effect of the factor on HAZ. In multivariable analysis, the final multivariable regression coefficient is adjusted for child age, sex and region (control variables) and all confounders in preceding levels.
Oaxaca-Blinder decomposition
Our Oaxaca-Blinder decomposition analysis is based largely on individual and household-level data, with larger sample sizes and higher statistical power. We focused on index mother-child pairs (i.e., the youngest child and youngest mother in any given household). This standard approach simplifies modeling and interpretation with minimal loss in data. Again, we analyzed the study period in each country and applied a similar hierarchical modeling approach as used in the regression approaches explained above.
We used height-for-age Z-score (HAZ) as the outcome of interest. The analysis was stratified by child age group where possible based on data availability (e.g. <6 months, 6-23 months, 24-59 months, all children under five).
Importantly, the Oaxaca-Blinder decomposition allowed us to identify the relative contribution of each predictive factor to HAZ change. We did this by using a linear least square regression model, accounting for survey design and weights, to assess associations between HAZ, time, control variables (i.e., child age and sex), and any trend effects.
Qualitative analysis
To investigate a diverse range of stakeholder perspectives (e.g. national experts, mothers, health care workers, etc.), we conducted a series of in-depth interviews with these stakeholders and held a set of focus group discussions with local actors, including community health workers and mothers.
The profiles of our national experts ranged from nutrition experts to representatives from donor organizations, ministries, and state agencies, among others. Respondents were asked to describe key nutrition-specific and -sensitive events in-country as well as comment on key trends and contextual factors that impacted child undernutrition over time. Community representatives, including health workers, health volunteers, and mothers, were also interviewed to provide community-level perspectives and experiences on transitions in nutrition and stunting.
Policy / program review & financing analysis
We conducted additional research to understand the implementation of policies, programs, and strategies during our study period, corroborating our findings with country experts. A similar multi-pronged data collection and corroboration exercise was undertaken to track financial data linked to our nutrition policy and program timeline.